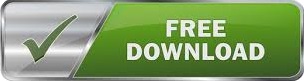
Garg S, Pal SK, Chakraborty D (2007) Evaluation of the performance of backpropagation and radial basis function neural networks in predicting the drill flank wear. Panda SS, Chakraborty D, Pal SK (2007) Monitoring of drill flank wear using fuzzy back-propagation neural network. Ramesh K, Alwarsamy T, Jayabal S (2015) Prediction of cutting process parameters in boring operations using artificial neural networks. Rao KV, Murthy BSN, Rao NM (2013) Cutting tool condition monitoring by analyzing surface roughness, work piece vibration and volume of metal removed for AISI 1040 steel in boring. Kaplan Y, Motorcu A, Nalbant M, Okay Ş (2015) The effects of process parameters on acceleration amplitude in the drilling of cold work tool steels. īalaji M, Murthy BSN, Rao NM (2016) Optimization of cutting parameters in drilling of Ti–6Al–4V Alloy using Taguchi and ANOVA. īalaji M, Murthy BSN, Rao NM (2016) Optimization of cutting parameters in drilling of AISI 304 stainless steel using Taguchi and ANOVA.
.jpg)
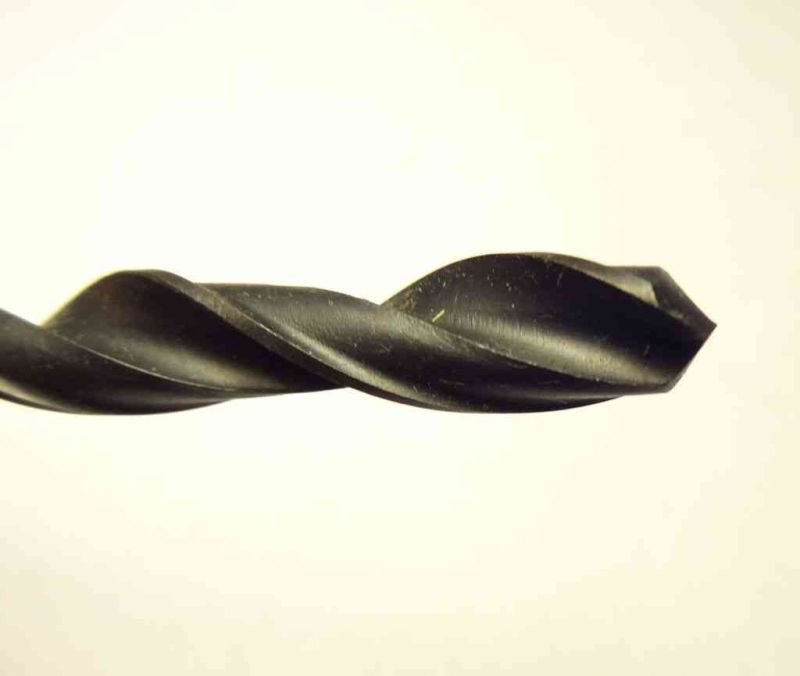
Novakov T, Jackson MJ (2009) Chatter in micro and macrodrilling. Mehrabadi IM, Nouri M, Madoliat R (2009) Investigating chatter vibration in deep drilling, including process damping and the gyroscopic effect. Īrvajeh T, Ismail F (2006) Machining stability in high speed drilling-Part 2: time domain simulation of a bending–torsional model and experimental validations. Īrvajeh T, Ismail F (2006) Machining stability in high-speed drilling-Part 1: modeling vibration stability in bending. Roukema JC, Altintas Y (2006) Time domain simulation of torsional–axial vibrations in drilling. Part II: chatter stability in frequency domain. Roukema JC, Altintas Y (2007) Generalized modeling of drilling vibrations. Part I: time domain model of drilling kinematics, dynamics and hole formation. Lin C, Kang SK, Ehmann KF (1995) Helical micro-drill point design and grinding. Yang Z et al (1998) Study for increasing micro-drill reliability by vibrating drilling. Park SS, Rahnama R (2010) Robust chatter stability in micro-milling operations. (03)00023-3Įrkki J (2002) A summary of methods applied to tool condition monitoring in drilling. Issam A-M (2003) Drilling wear detection and classification using vibration signals and artificial neural network. (03)00148-1Įma S, Fujii H, Marui E (1988) Chatter vibration in drilling.

Įma S, Marui E (2003) Theoretical analysis on chatter vibration in drilling and its suppression. Ĭhern G-L, Lee H-J (2006) Using workpiece vibration cutting for micro-drilling. Guillem Q, Ciurana J (2011) Chatter in machining processes: a review. In this context, high-reliability ANN model has been developed with a 4 input (cutting speeds, feed rates, cutting tool type and time) and 3 output ( x, y, and z vibration values). The aim of the study is to ensure the simulation of the vibrations that may occur during hole drilling processes via a model. Secondly, input and output parameters are determined by classifying the data obtained in the experimental work, then a new ANN model is developed, and the results are compared with the experimental data. An experimental setup for vibration measurement is prepared so that the technical equipment works in harmony with each other. Firstly, the hole drilling operation is applied to C2080 (AISI D3) cold work tool steel workpiece with high-speed steel and carbide cutting tools at cutting speeds of 15, 20, 25 and 30 m/min and at feed rates of 0.06, 0.08, 0.1 and 0.12 mm/(rev) and the vibrations in the x, y and z axes are measured. Drilling tests (total of 1304 experiments) are performed experimentally and modeled with artificial neural networks (ANN). The effects of these vibrations on the cutting tool and material are investigated. In order to minimize these effects, an experimental study is conducted and the vibrations generated during the process are measured.

These vibrations adversely affect cutting tool’s life span, the measurement accuracy of the workpiece and the surface quality. Vibrations occur in the cutting tool during machining.
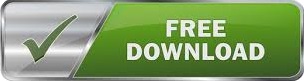